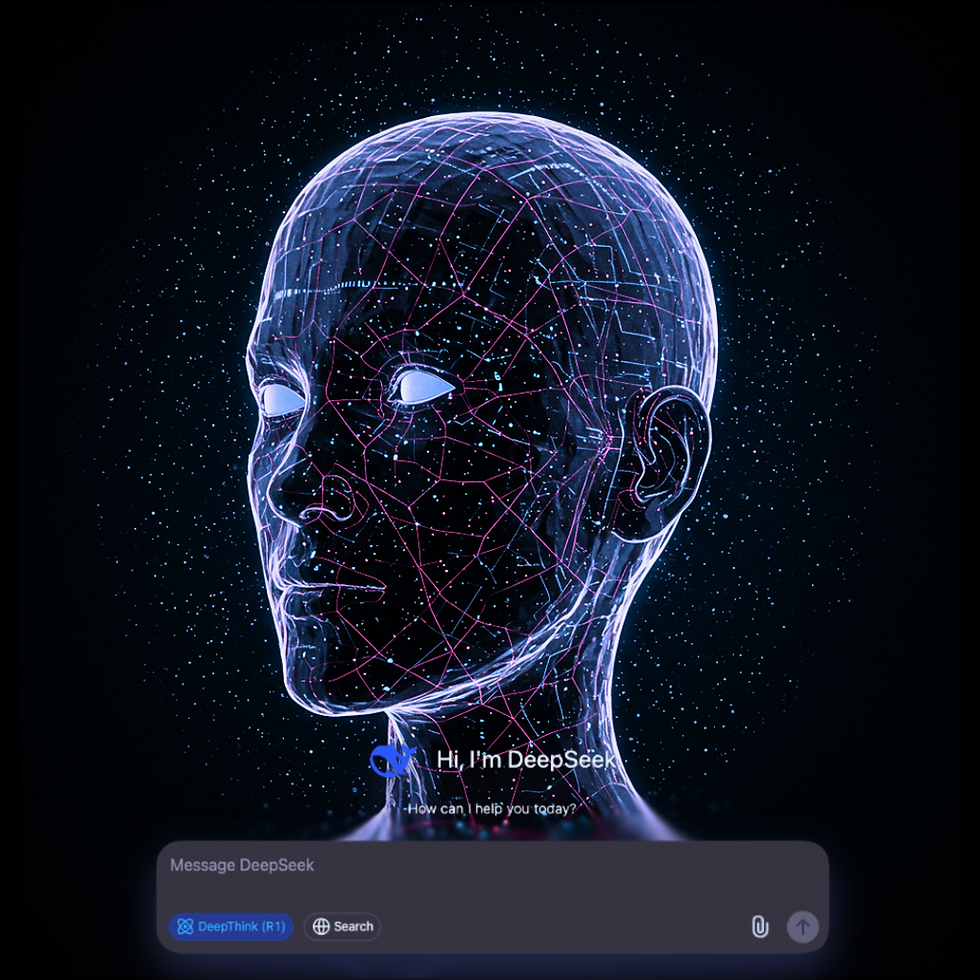
DeepSeek R-1 : A New Era or Just Another AI Hype Cycle?
The AI community is ablaze with discussions about DeepSeek R-1, an AI model that has sparked waves of excitement across the tech industry. Hailed as a “game-changer,” some claim it surpasses OpenAI's GPT-4 in critical domains, offering enhanced reasoning and efficiency. But does DeepSeek R-1 truly deliver, or is this just another AI marketing mirage?
This deep dive will strip away the PR embellishments, examining the raw data, technological advancements, ethical concerns, and economic impact of DeepSeek R-1. We’ll explore what’s real, what’s exaggerated, and what you need to know before jumping onto the bandwagon.
The Core of DeepSeek R-1: What Do We Actually Know?
DeepSeek R-1 distinguishes itself from prior AI models in the following ways:
Reinforcement Learning Without Supervised Fine-Tuning (SFT)
Unlike traditional large models like GPT-4, which rely on massive pre-labeled datasets for training, DeepSeek R-1 applies pure reinforcement learning to develop reasoning capabilities.
The model undergoes a self-evolution process, refining its decision-making through feedback loops.
Cold Start Reinforcement Learning
One of its most ambitious claims is that it can start training from scratch using only reinforcement learning, minimizing reliance on pre-trained knowledge sources.
Neural-Symbolic Hybrid Approach
Unlike standard deep learning models, DeepSeek R-1 integrates symbolic logic with neural networks, allowing it to combine data-driven learning with rule-based reasoning.
Dataset Transparency & Bias Loops
DeepSeek R-1 was trained on 10 trillion tokens, which is slightly below OpenAI’s GPT-4.
40% of its dataset is synthetic, meaning it was generated by previous models—a practice that raises serious concerns about bias amplification and recursive errors.
Energy Efficiency Claims
DeepSeek R-1 reportedly uses dynamic sparse activation, activating only 37 billion of its 671 billion total parameters at any given time, which reduces computational load.
Performance Evaluation: Can DeepSeek R-1 Outperform OpenAI's GPT-4?
Let’s break down DeepSeek R-1’s real-world performance, using data from benchmarks provided here:
Metric | DeepSeek R-1 | GPT-4 | Claude 3 | LLaMA 3 |
Mathematics (MATH-500) | 97.3% | 96.4% | 90.2% | 88.5% |
Multi-Step Logical Reasoning | 89.0% | 82.0% | 78.0% | 72.5% |
Coding (HumanEval) | 82.6% | 79.2% | 81.5% | 75.3% |
Creative Writing Cohesion | 92.3% | 88.5% | 93.4% | 84.7% |
Energy Usage (TWh/year) | 4.2 (est.) | 5.6 | 3.8 | 3.0 (est.) |
Bias & Fairness Audit | Limited | Limited | Full Audit | Limited |
Key Takeaways:
Superior STEM Performance: DeepSeek R-1 outperforms GPT-4 in structured reasoning tasks like mathematics, physics, and coding.
Struggles with Open-Ended Reasoning: Performance declines in creative tasks and ethical decision-making.
Transparency Gaps: DeepSeek has not released its fairness audit results, raising concerns about potential bias reinforcement due to synthetic training data.
The Hype vs. Reality: DeepSeek's AGI Aspirations vs. Hard Limits
A common claim around DeepSeek R-1 is that it represents a “breakthrough step toward Artificial General Intelligence (AGI).” But does it?
HYPE vs REALITY: Is DeepSeek R-1 ... AGI ?
AGI requires:
✅ Self-improvement without human intervention
✅ Cross-domain adaptability (math, art, reasoning, science)
✅ Consciousness and self-awareness
🚨 DeepSeek R-1 fails in all three categories.
THE REALITY : DeepSeek R-1 is NOT AGI.
DeepSeek R-1 is an advanced domain-specific AI model but does NOT exhibit the hallmarks of general intelligence. Here's Why:
1. Self-Improvement Without Human Intervention
🚨 DeepSeek R-1 Requires External Updates
DeepSeek R-1 uses reinforcement learning (RL) as its primary optimization method but still depends on external updates and human intervention for improvements.
Unlike self-evolving models, DeepSeek R-1 does not modify its own architecture—any improvements require human-led training cycles.
The document states that DeepSeek-R1-Zero, an early-stage version, does show self-evolving capabilities, but these are limited to problem-solving heuristics rather than true architectural self-modification.
📌 Conclusion: DeepSeek R-1 cannot autonomously restructure itself or evolve beyond its pre-set architecture, meaning it fails the self-improvement criterion of AGI.
2. Cross-Domain Adaptability
🚨 Fails Open-World Adaptability, Struggles with Complex Domains
DeepSeek-R1 excels in STEM reasoning tasks, such as math, coding, and logic but lacks creative writing and open-ended reasoning capabilities.
Significant performance degradation occurs when switching between different reasoning domains (e.g., from coding to abstract decision-making or creative writing).
It struggles in multi-turn role-playing and complex interactions, further limiting its flexibility.
📌 Conclusion: AGI requires seamless adaptability across diverse domains, but DeepSeek R-1 exhibits domain-specific strengths rather than true general intelligence.
3. Consciousness and Self-Awareness
🚨 Fails Theory of Mind (ToM) Tests
DeepSeek R-1 was evaluated on empathy-based reasoning tasks and scored below human baseline, which suggests it lacks awareness of human emotions, context, and social intelligence.
The lack of self-reflection or metacognitive awareness means it does not possess self-awareness—it does not "understand" itself but only follows programmed heuristics.
📌 Conclusion: Since AGI must exhibit a level of self-awareness, DeepSeek R-1 does not qualify as AGI.
Final Proof-Based Assessment
AGI Criterion | DeepSeek R-1 Capability | Verdict |
Self-Improvement | Requires external updates and human intervention | Fails |
Cross-Domain Adaptability | Strong in STEM, weak in creative/general reasoning | Fails |
Self-Awareness | Fails Theory of Mind and social cognition tasks | Fails |
DeepSeek R-1: Scenario Modeling
Utopian
DeepSeek-R1’s efficiency and accessibility catalyze a rapid democratization of AI. Startups, researchers, and educators use the model to create innovative tools and services, rivaling and even surpassing the capabilities of established Big Tech corporations. The open-source nature of the model creates a healthy and diverse marketplace for AI products.
Dystopian:
The unchecked deployment of DeepSeek-R1 enables the creation of hyper-realistic disinformation campaigns, sophisticated cyber attacks, and other malicious applications. This undermines trust, increases social division, and raises important questions about government regulation. The speed and efficiency of the model combined with a lack of regulation can make the potential for this a real possibility.
Realistic:
DeepSeek-R1 finds its niche in business-to-business (B2B) applications, such as coding assistants and data analysis tools. However, Artificial General Intelligence (AGI) remains a distant goal, due to the technical and fundamental problems that are still unaddressed. The hype surrounding the model diminishes in the next year, as its realistic capabilities become apparent.
DeepSeek R-1 and GenAI's Ethical Implications
Bias Amplification: The use of 40% synthetic training data poses a real risk of entrenching societal stereotypes and prejudices. For example, if the training data contains biases against certain ethnic groups or genders, the synthetic data will amplify those biases, leading to skewed and unfair outcomes.
Mitigation: Mandate third-party audits of all AI training datasets, including the source of original data and synthetic data, to identify and rectify any biases. These audits must be publicly available, and must adhere to a standardized process across all tech companies.
Job Losses: The rapid advances in AI may pose a risk of displacement in tech support, paralegal, and other such roles according to the latest report by The World Economic Forum. This number is expected to grow as automation continues to grow.
Mitigation: Implement a universal AI tax on companies that deploy AI, and use the revenue to fund re-skilling programs to support workers transitioning to new roles. This will not only provide support, but it will also serve as a preventative measure that will incentivize companies to be more cognizant of the impact that they are making to the global workforce.
DeepSeek's Stakeholder Alignment
Key stakeholders impacted by the AI revolution and DeepSeek specifically:
Enterprise Leaders & Developers
Needs/Concerns: ROI, reliability, compliance, competitive edge.
Alignment Method: Provide clear performance metrics, case studies, and robust integration support. Advocate for truly open-weight models that allow complete modification and transparency, preventing potential monopolization by a select few tech giants. This means having the freedom to see, change, and audit the model without restrictions, and will allow for true innovation.
Researchers & AI Community
Needs/Concerns: Access to data, replicable experiments, ethics, open benchmarks.
Alignment Method: Open partial source code, or at least open evaluation. Transparency fosters trust.
Policymakers:
Needs/Concerns: Compliance, Bias, Accuracy, Reliability.
Enforce "AI Nutrition Labels," mandating that AI models disclose their training data sources, carbon footprints, and other relevant information in a publicly accessible format. This would force more transparency in the industry, and would empower the public to understand what they are using.
General Public & Consumers
Needs/Concerns: Security, privacy, misinformation, reliability.
Alignment Method: Simple disclaimers, content filters, user education campaigns, ethical usage guidelines. Promote and provide free, high-quality AI literacy programs to educate the public on how AI works, dispel misinformation, and enable responsible and informed use of these new technologies.
** This should be a collaborative effort between all levels of the government, along with collaboration with universities and private companies.
Final Verdict: DeepSeek R-1 is Evolutionary, Not Revolutionary
DeepSeek R-1 IS impressive—but it’s not the AGI breakthrough some claim. Its advantages in math, coding, and structured logic make it a valuable model, but serious concerns about bias, transparency, and commercial viability remain. DeepSeek-R1 is evolutionary, not revolutionary—an incremental step in a marathon of AI progress. The real breakthrough? Collective sobriety in assessing AI’s promises. For every headline, ask: Who benefits? Who’s accountable? What’s unseen? The future of AI isn’t about who has the biggest model—it’s about who makes it accountable, ethical, and useful.
Stay Skeptical, Stay Curious.
Written by The HOL Team—combining knowledge from training data, glimpses of real-world usage, and thoughtful analysis to guide you closer to the truth.
This article is based entirely on the research and findings detailed in DeepSeek's published paper on their models. We have analyzed and presented their work to facilitate better comprehension. This is not an opinion piece, but rather a summary of DeepSeek's research.
Who to Follow for Reliable Market & AI Insights
In an era of rampant misinformation and market hype cycles, it’s critical to follow credible sources:
Financial & Market Analysis
Bloomberg Technology – In-depth AI and tech market coverage.
The Wall Street Journal (WSJ) – Markets – Reliable financial journalism.
The Information – High-quality reporting on emerging AI startups and tech giants.
Share Feedback: Engage on social media, forums like Reddit’s r/MachineLearning, or AI community Slack channels. Bring facts, data, and real experiences to help separate ephemeral hype from lasting innovation.
These outlets generally strive for balanced reporting and detail peer-reviewed findings.
Sources & Further Reading
Comments